===============================
학습내용
(3강) Recurrent Neural Network and Language Modeling
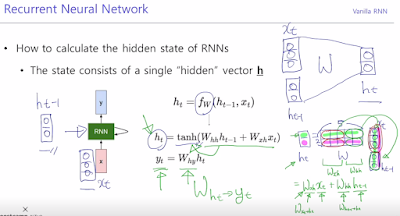
W는 t와 관계없이 어디에서나 같다. 입력인 xt와 ht-1 을 한 벡터로 연결하고 그걸 계산하는 W 로 생각할 수도 있고 나눠서 생각할 수도 있다. 현재 t 시간에서 출력값이 필요할 경우 W(hy)로 출력값 yt 만들 수 있음.
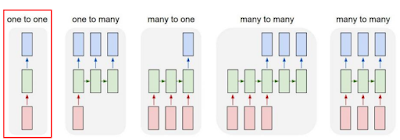
one to many의 경우 우린 지금까지 xt가 계속 있는걸로 배웠기 때문에 x에 입력값 0을 넣어주는 방식으로 한다.
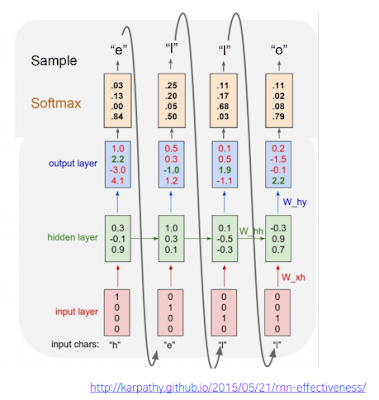
이런식으로 피드백모델. 위에서 입력 "h"의 경우 target은 "e"지만 결과는 "o" 가 나온걸 알 수 있다. 그래서 loss func을 "e" 가 나오도록 잘 정의해야겠지.
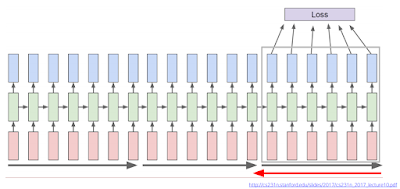
모든 것을 하나의 loss에 두기엔 메모리가 부족하다. 그래서 저렇게 나눠서 학습하기도 한다.
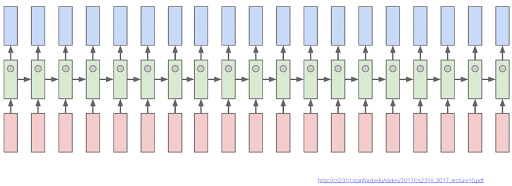
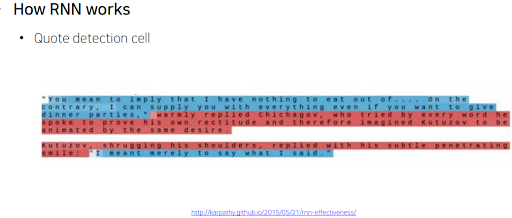
이런식으로 기억 소자도 생긴다. 빨간색일수록 +1, 파란색일수록 -1이고 hidden state를 나타냄.
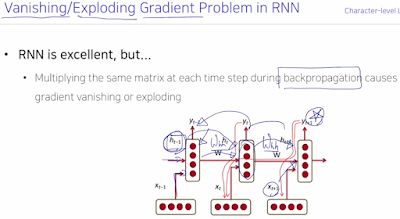
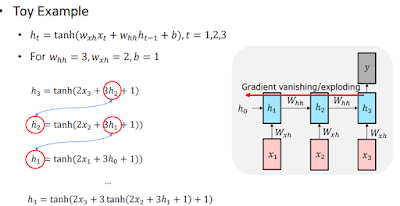
RNN 의 문제라면 계속 같은 W를 곱해주기 때문에 weight 값이 폭발하거나 사라지는 현상이 생긴다. 왜 그러나면 앞의 계수가 1 이 넘으면 계속 곱해주다 보니 폭발하고 1보다 작으면 사라진다. 위 그림에서 h3의 경우 h1에 대한 편미분을 하려고 보면 앞에 붙어있는 3을 계속 곱한걸 계산하게 되고 결과적으로 엄청커짐. 그래서 LSTM을 쓴다. 계수가 절대값 1 미만일때 밑의 그림을 보면 RNN은 금방 vanishing 되지만 LSTM은 오랫동안 살아있음.
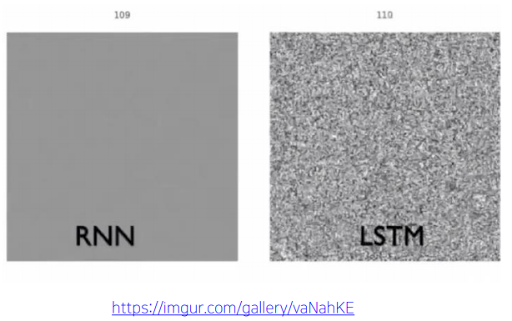
(4강) LSTM and GRU
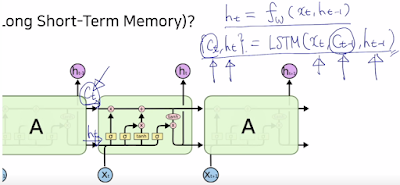
xt와 ht-1을 받아 ht가 나온 vanilla RNN 과 달리 xt, Ct-1, ht-1 을 받아 Ct 와 ht를 내놓는다.
Ct에는 중요한 여러 정보를 가지고 있고 ht-1, xt와 결합해서 같이 계산한다.
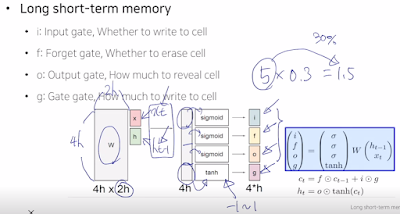
만약 x, h 둘다 크기가 h라고 하면, 두개 다 이어붙이기 때문에 W 열은 2h가 되고, 4개의 필터를 사용할 거라 4h 크기의 행을 가지게 된다. 각각을 h 크기로 나눠 필터로 사용할 거다. 그래서 필터는 Input gate, Forget gate, Output gate, Gate gate 4개가 있는데, i, f, o는 sigmoid를 사용해서 0~1의 값을 가지고 g는 tanh 를 사용해 -1~1의 값을 가진다. 그래서i, f, o는 얼마나 값을 가지고 갈지 배율을 통해 결정하고, g는 tanh를 사용하는게 RNN에서 선형 hidden state를 사용해 -1~1의 값을 가지게 해서 의미를 가져서 유의미한 정보를 가지게 해서 사용하는 것 같다.
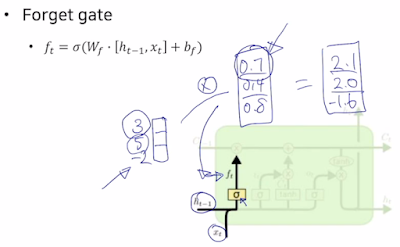
Forget gate는 가지고 있던 ht-1에서 xt와 결합해 softmax로 0~1 값을 가지게 한다. 그래서 나온 gate 결과와 ht-1를 곱해서 나온 값으로 어느 값을 얼마나 잊어버릴 지 정한다.
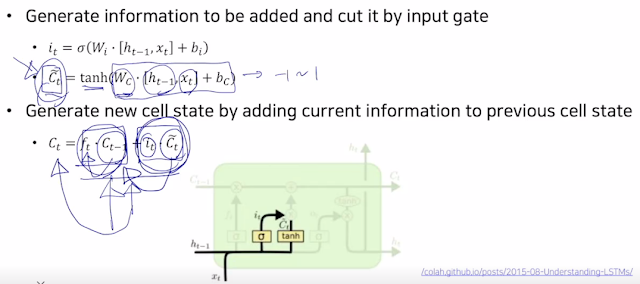
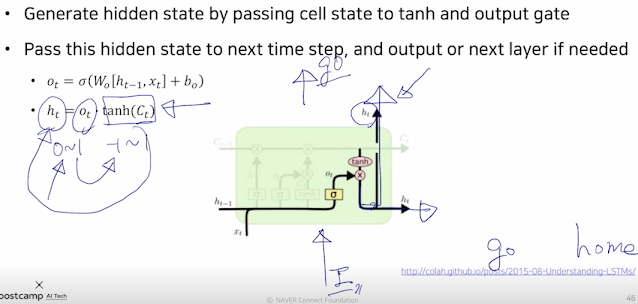
output 이 필요하다면 계산된 Ct를 가지고 현재 계산을 내놓는다.
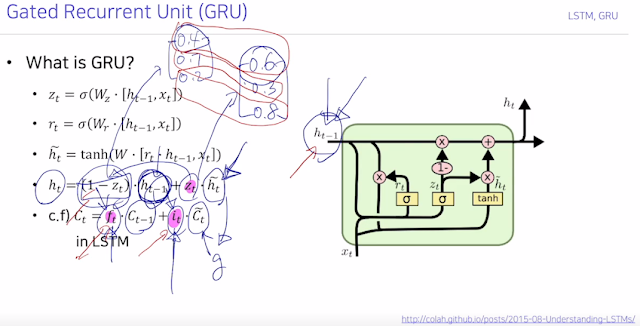
GRU는 위 LSTM의 단점인 메모리를 많이 먹는 것과 계산할게 많다는 점을 보완하기 위해 나온 것. Ct와 ht를 그냥 ht로 합치고 LSTM에서 Input gate와 Forget gate를 하나의 zt 값으로 만들어서 계산한다. 확실히 가볍고 빠르긴 한데 LSTM보다 잘 될때도 있고, 안될때도 있다고 한다.
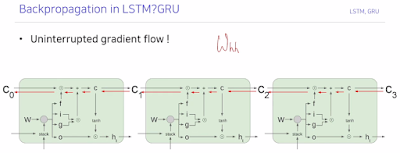
실습
pack_padded_sequence, pad_packed_sequence 을 어떻게 쓰는지
language token 은 순차적으로 token을 만드는것. 원래 목적이 앞으로 어떤 단어를 input으로 넣을지 모르는데 다음 단어를 예측하는 거니까.
근데 여기서 본 force tagging 이나 entity recognition는 이미 모든 token을 알고 수행하는 것. rnn이 각각의 token을 알아서 넣어주는 것. 즉 rnn 자체가 rnn cell 하나를 문장 길이만큼 for문으로 호출하는 class가 수행하는 거라고 보면 된다. 근데 실제로 language model은 그렇지 않으니까 직접 for문을 돌아 각 rnn 셀에 한 단어씩 input을 넣어주고 그렇게 나온 결과를 다시 input으로 정해서 넣어주는 과정을 구현해줘야 함.
##3. RNN 1. 주어진 데이터를 RNN에 넣을 수 있는 형태로 만듭니다. 2. 기본적인 RNN 사용법 및 적용법을 익힙니다. 3. PackedSquence의 필요성에 대해 배우고 적용법을 실습합니다.
필요 패키지 import
데이터 전처리
아래의 sample data를 확인해봅시다.
전체 단어 수와 pad token의 id도 아래와 같습니다.
Padding 처리를 해주면서 padding 전 길이도 저장합니다.
위 데이터를 하나의 batch로 만들어 실습에 이용하겠습니다.
RNN 사용해보기
RNN에 넣기 전 word embedding을 위한 embedding layer를 만듭니다.
아래와 같이 RNN 모델 및 초기 hidden state를 정의합니다.
RNN에 batch data를 넣으면 아래와 같이 2가지 output을 얻습니다.
- hidden_states: 각 time step에 해당하는 hidden state들의 묶음.
- h_n: 모든 sequence를 거치고 나온 마지막 hidden state.
RNN 활용법
마지막 hidden state를 이용하여 text classification task에 적용할 수 있습니다.
각 time step에 대한 hidden state를 이용하여 token-level의 task를 수행할 수도 있습니다.
PackedSequence 사용법
앞서 padding 처리했던 데이터를 다시 확인해봅시다.
[[85, 14, 80, 34, 99, 20, 31, 65, 53, 86, 3, 58, 30, 4, 11, 6, 50, 71, 74, 13],
[62, 76, 79, 66, 32, 0, 0, 0, 0, 0, 0, 0, 0, 0, 0, 0, 0, 0, 0, 0],
[93, 77, 16, 67, 46, 74, 24, 70, 0, 0, 0, 0, 0, 0, 0, 0, 0, 0, 0, 0],
[19, 83, 88, 22, 57, 40, 75, 82, 4, 46, 0, 0, 0, 0, 0, 0, 0, 0, 0, 0],
[70, 28, 30, 24, 76, 84, 92, 76, 77, 51, 7, 20, 82, 94, 57, 0, 0, 0, 0, 0],
[58, 13, 40, 61, 88, 18, 92, 89, 8, 14, 61, 67, 49, 59, 45, 12, 47, 5, 0, 0],
[22, 5, 21, 84, 39, 6, 9, 84, 36, 59, 32, 30, 69, 70, 82, 56, 1, 0, 0, 0],
[94, 21, 79, 24, 3, 86, 0, 0, 0, 0, 0, 0, 0, 0, 0, 0, 0, 0, 0, 0],
[80, 80, 33, 63, 34, 63, 0, 0, 0, 0, 0, 0, 0, 0, 0, 0, 0, 0, 0, 0],
[87, 32, 79, 65, 2, 96, 43, 80, 85, 20, 41, 52, 95, 50, 35, 96, 24, 80, 0, 0]]
아래 그림과 같이 불필요한 pad 계산이 포함됩니다.
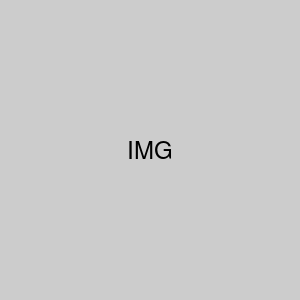
데이터를 padding전 원래 길이 기준으로 정렬합니다.
아래와 같은 padding 무시 효과를 얻을 수 있습니다.
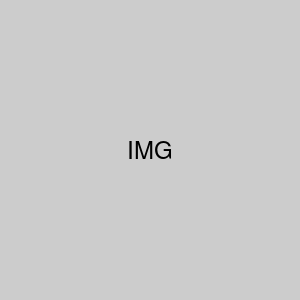
pack_padded_sequence를 이용하여 PackedSquence object를 사용합니다.
packed_output은 PackedSquence이므로 원래 output 형태와 다릅니다.
이를 다시 원래 형태로 바꿔주기 위해 pad_packed_sequence를 이용합니다.
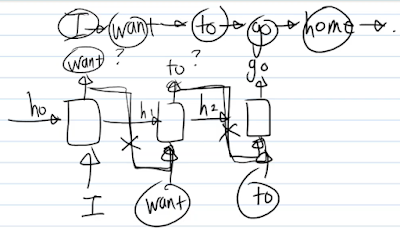
teacher forcing 은 맨 처음 학습할 땐 맞추는게 불가능에 가까운데 그렇게 틀린 결과를 다음 input에 넣고 또 틀린걸 다음 input에 넣고... 하니까 끝이 없고 그냥 개판난다. 그래서 처음엔 연결을 끊고 알맞게 출력했다고 가정하며 input으로 넣는 cheating을 해줌. 그 다음부터는 지가 어찌어찌 알아서 잘 해주겠지.
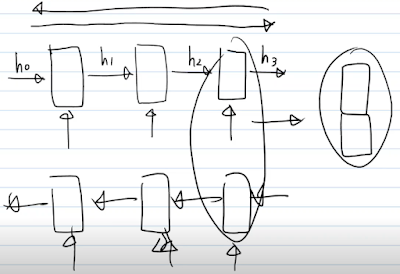
##4. LSTM, GRU 1. 기존 RNN과 다른 부분에 대해서 배웁니다. 2. 이전 실습에 이어 다양한 적용법을 배웁니다.
필요 패키지 import
데이터 전처리
아래의 sample data를 확인해봅시다.
이전 실습과 동일합니다.
LSTM 사용
LSTM에선 cell state가 추가됩니다.
Cell state의 shape는 hidden state의 그것과 동일합니다.
GRU 사용
GRU는 cell state가 없어 RNN과 동일하게 사용 가능합니다.
GRU를 이용하여 LM task를 수행해봅시다.
Teacher forcing 없이 이전에 얻은 결과를 다음 input으로 이용합니다.
max_len만큼의 for 문을 돌면서 모든 결과물의 모양을 확인했지만 만약 종료 조건(예를 들어 문장의 끝을 나타내는 end token 등)이 되면 중간에 생성을 그만둘 수도 있습니다.
양방향 및 여러 layer 사용
이번엔 양방향 + 2개 이상의 layer를 쓸 때 얻을 수 있는 결과에 대해 알아봅니다.
Bidirectional이 되었고 layer의 개수가 22로 늘었기 때문에 hidden state의 shape도 (4, B, d_h)가 됩니다.
각각의 결과물의 shape는 다음과 같습니다.
outputs: (max_len, batch_size, num_dir * hidden_size)
h_n: (num_layers*num_dirs, batch_size, hidden_size)
=============================
과제 / 퀴즈
Natural Language Processing
Assignment 2: 번역 모델 전처리
0. Introduction
- 본 과제의 목적은 영어-한글 번역 모델을 학습하기 위해 영어-한글 번역 데이터셋을 전처리하는 방법을 학습하는 것입니다. 이번 과제에서는 번역 모델의 입/출력을 만들기 위해 자주 사용되는 여러가지 자연어 전처리 기술을 익히게 됩니다. 번역 모델은 번역하고자 하는 문장(Source)을 입력으로 받고, 번역 결과(Target)을 출력합니다.
- 미완성된 함수 3개가 있습니다. (preprocess, collate_fn, bucketing) 아래의 지시 사항과 각 함수의 docstring을 참고하여 함수 3개를 완성하여 Data Loader를 완성해주세요. test case를 모두 통과해야 합니다.
1. Sentence Preprocessor
- NLP 모델에 자연어 정보를 전달하기 위해서는 적절한 형태로의 전처리가 필요합니다. 주어진 데이터셋은 Source, Target(한->영 번역의 경우 source는 한국어 문장, target은 영어 문장이 됩니다.) 각각 하나의 문장으로 이루어져 있고 모델에 해당 정보를 전달하기 위해서는 하나의 문장을 여러 단어로 분리하고 각각의 단어를 index로 바꿔줄 수 있는 word2idx dictionary가 필요합니다(동일한 단어 = 동일한 index).
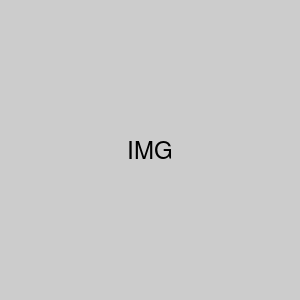
해당 과정은 가장 간단한 수준의 tokenization이며 거의 모든 자연어 전처리 과정에서 사용됩니다. 주어진 문장쌍(Source, Target)을 단어 index 단위로 바꾸어주는 preprocess 함수를 docstring을 참고하여 완성해주세요.
- 추가사항: 번역 모델에서 Target 문장에는 sos(start of sentence), eos(end of sentence) token이 추가되고 각각은 문장의 시작과 끝을 알려주는 token으로 사용됩니다. (그림 참고)
1-1. Preprocess 테스트 케이스 확고
2. Bucketing
- Bucketing은 주어진 문장의 길이에 따라 데이터를 그룹화하여 padding을 적용하는 기법입니다. 이 기법은 모델의 학습 시간을 단축하기 위해 고안되었습니다. 아래 그림과 같이 bucketing을 적용하지 않은 경우, batch별 pad token의 개수가 늘어나 학습하는 데에 오랜 시간이 걸립니다. 주어진 문장들을 문장 길이를 기준으로 나누어 bucketed_batch_indices 함수를 완성해주세요.
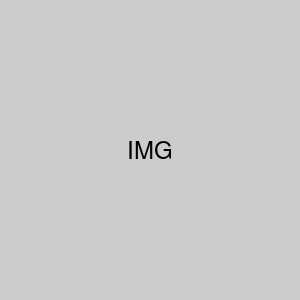
Figure 1. Bucketing을 적용하지 않은 경우
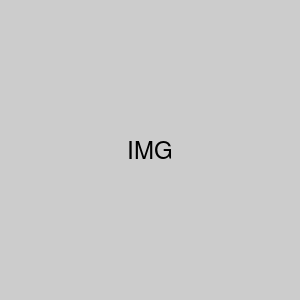
Figure 2. Bucketing을 적용한 경우
2-1. Bucketing 테스트 케이스 확인
3. Collate Function
- Collate function은 주어진 데이터셋을 원하는 형태의 batch로 가공하기 위해 사용되는 함수입니다. Batch 단위별로 max sequence length에 맞게 pad token을 추가하고 내림차순으로 정렬하는 collate_fn 함수를 완성해주세요.
3-1. Collate function 테스트 케이스 확인
3-2. 전처리 테스트 케이스 확인
==========================
피어세션
그냥 저저번주 금요일꺼부터 복습해봄.
======================